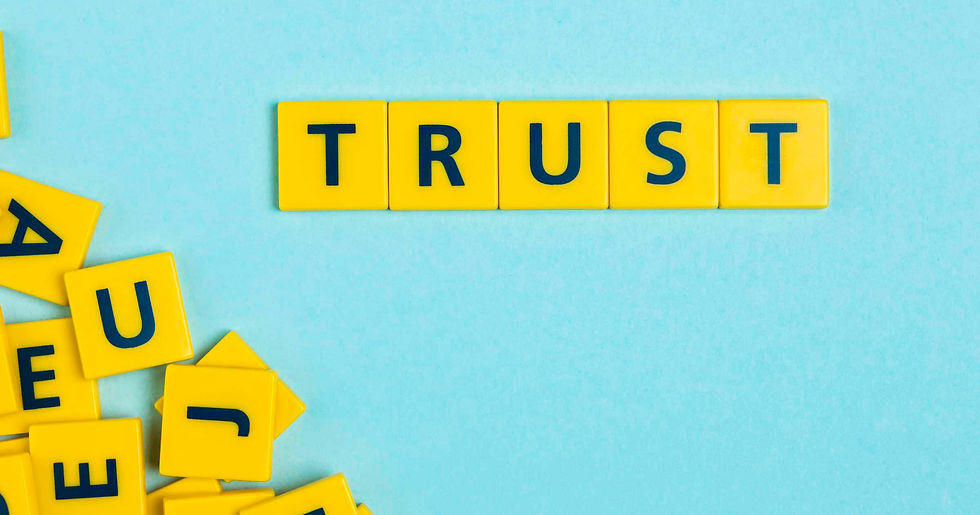
Introduction
In the age of big data, transparency in data analytics is paramount. It not only enhances the credibility of the insights generated but also fosters trust among stakeholders.
This article delves into the importance of transparency in data analytics and explores practical ways to achieve it.
Importance of Transparency in Data Analytics
1.Trust Building: Transparency fosters trust among stakeholders, including clients, customers, and regulatory bodies. When the processes and methodologies are transparent, stakeholders feel more confident in the accuracy and reliability of the data insights.
2. Ethical Compliance: Transparent practices ensure that data analytics adhere to ethical standards. This includes proper data collection, processing, and reporting, which is crucial in maintaining public trust and avoiding legal repercussions.
3. Accountability: Clear documentation and openness in methodologies make it easier to hold individuals or teams accountable for their actions and decisions. This is crucial in identifying and rectifying errors or biases in the analytics process.
4. Enhanced Collaboration: When data analytics processes are transparent, it promotes better collaboration among teams. Team members can easily understand and contribute to the project, leading to more robust and accurate outcomes.
Achieving Transparency in Data Analytics
1.Clear Documentation: Maintain comprehensive documentation of all stages of the data analytics process. This includes data sources, methodologies, algorithms used, and the rationale behind key decisions. Proper documentation serves as a reference point for future audits and reviews.
2. Open Communication: Foster a culture of open communication within the organization. Encourage data scientists and analysts to share their processes, challenges, and findings with stakeholders. Regular updates and reports can help in keeping everyone informed and aligned.
3. Data Provenance: Implement data provenance practices to track the origin and transformations of data. This involves keeping a detailed record of where the data comes from, how it has been processed, and any changes made. This helps in verifying the accuracy and reliability of the data used.
4. Explainable AI and Models: Use explainable AI techniques to make complex models understandable to non-technical stakeholders. Providing explanations for how models make decisions can demystify the analytics process and build trust in the results.
5. Ethical Guidelines: Establish and adhere to ethical guidelines for data analytics. This includes obtaining proper consent for data collection, ensuring data privacy, and avoiding biases in data processing and analysis. Ethical practices are foundational to building trust.
6. Third-Party Audits: Engage independent third-party auditors to review and validate the data analytics processes and results. External audits can provide an unbiased assessment and enhance credibility.
7. Transparency Tools and Platforms: Utilize tools and platforms that enhance transparency. Tools like data visualization platforms can help in presenting data and insights in an easily understandable manner. Transparency platforms can provide real-time tracking and reporting of analytics processes.
Challenges to Achieving Transparency
1.Complexity of Data Processes: The intricate nature of data analytics processes can make transparency challenging. Complex algorithms and large datasets may be difficult to explain in simple terms.
2. Data Privacy Concerns: Balancing transparency with data privacy can be tricky. While stakeholders need to understand the processes, sensitive information must be protected.
3. Resource Constraints: Ensuring transparency requires additional resources in terms of time, effort, and cost. Organizations may face challenges in allocating sufficient resources for comprehensive documentation and audits.
Conclusion
Transparency in data analytics is not just a best practice; it is essential for building trust and ensuring the ethical use of data. By implementing clear documentation, fostering open communication, ensuring data provenance, utilizing explainable AI, adhering to ethical guidelines, engaging in third-party audits, and leveraging transparency tools, organizations can achieve greater transparency in their data analytics processes.
While challenges exist, the benefits of transparency far outweigh the costs, leading to more credible, reliable, and trustworthy data insights. For those looking to deepen their understanding of these practices, enrolling in a data analytics training course in Delhi , Noida and other locations in India can provide valuable knowledge and skills.
Comments