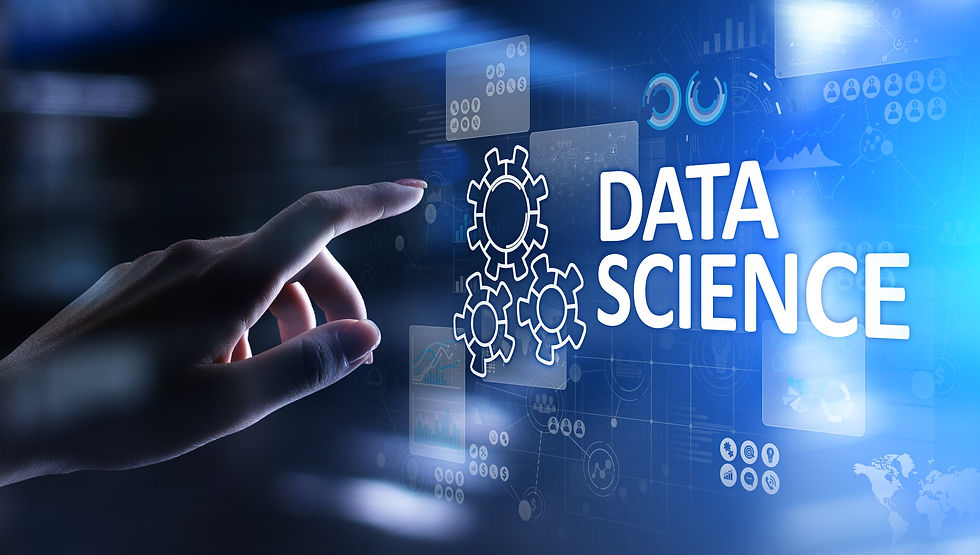
Introduction
The rapid advancements in automated data science tools have revolutionized the way businesses and researchers handle data. These tools promise to streamline workflows, enhance efficiency, and democratize access to data science capabilities.
However, alongside these opportunities come significant risks, including job displacement and over-reliance on automation.
This article explores the potential benefits and challenges posed by automated data science.
Opportunities
1. Increased Efficiency and Productivity
Automated data science tools can handle repetitive tasks, allowing data scientists to focus on more complex and creative aspects of their work.
These tools can process large volumes of data quickly, enabling faster insights and decision-making.
2. Accessibility and Democratization
Automated tools lower the barrier to entry for individuals and organizations without extensive data science expertise.
Small businesses and nonprofits can leverage these tools to gain insights from their data without needing to hire expensive data science teams.
3. Enhanced Accuracy and Consistency
Automation reduces the risk of human error in data processing and analysis.
Standardized processes ensure consistent application of best practices and methodologies.
4. Scalability
Automated tools can easily scale to handle growing data volumes and complexities.
Organizations can adapt to increasing data demands without proportionally increasing their workforce.
Risks
1. Job Displacement
The rise of automated tools may reduce the demand for traditional data science roles, leading to job displacement.
Workers may need to reskill or upskill to remain relevant in an increasingly automated job market.
2. Over-Reliance on Automation
Excessive dependence on automated tools can lead to a lack of critical thinking and oversight.
Organizations may blindly trust automated outputs without understanding the underlying processes and potential biases.
3. Bias and Fairness Issues
Automated tools can inadvertently perpetuate existing biases present in the training data.
Ensuring fairness and ethical use of automated data science requires careful monitoring and intervention.
4. Security and Privacy Concerns
Automated tools handling sensitive data may be vulnerable to security breaches and privacy violations.
Organizations must implement robust security measures to protect data integrity and confidentiality.
5. Loss of Human Touch
Automation may overlook nuanced insights that a human analyst could identify.
The human element in data interpretation and storytelling remains crucial for deriving meaningful conclusions.
Balancing Opportunities and Risks
To harness the full potential of automated data science while mitigating associated risks, organizations should adopt a balanced approach:
1. Integrating Human Expertise
Combine automated tools with human expertise to ensure robust and accurate data analysis.
Encourage collaboration between data scientists and automated systems to leverage the strengths of both.
2. Continuous Learning and Adaptation
Invest in ongoing training and development programs for employees to stay updated with the latest tools and techniques.
Foster a culture of continuous learning and innovation within the organization.
3. Ethical and Responsible Use
Implement ethical guidelines and frameworks for the use of automated data science tools.
Regularly audit and review automated processes to ensure fairness, transparency, and accountability.
4. Robust Security Measures
Prioritize data security and privacy by implementing advanced encryption and protection protocols.
Regularly update and monitor security systems to safeguard against potential threats.
Conclusion
Automated data science tools offer significant opportunities to enhance efficiency, accessibility, and scalability in data analysis. However, organizations must carefully navigate the associated risks, such as job displacement, over-reliance on automation, and ethical concerns. By integrating human expertise, fostering continuous learning through programs like data science training course in Delhi, Noida, and other locations in India, and adopting ethical practices, organizations can successfully leverage automated data science to drive innovation and informed decision-making in the future.
Comments