Integrating AI and ML with Data Analytics: Enhancing Capabilities and Transforming Insights
- shivanshi singh
- Jul 9, 2024
- 3 min read
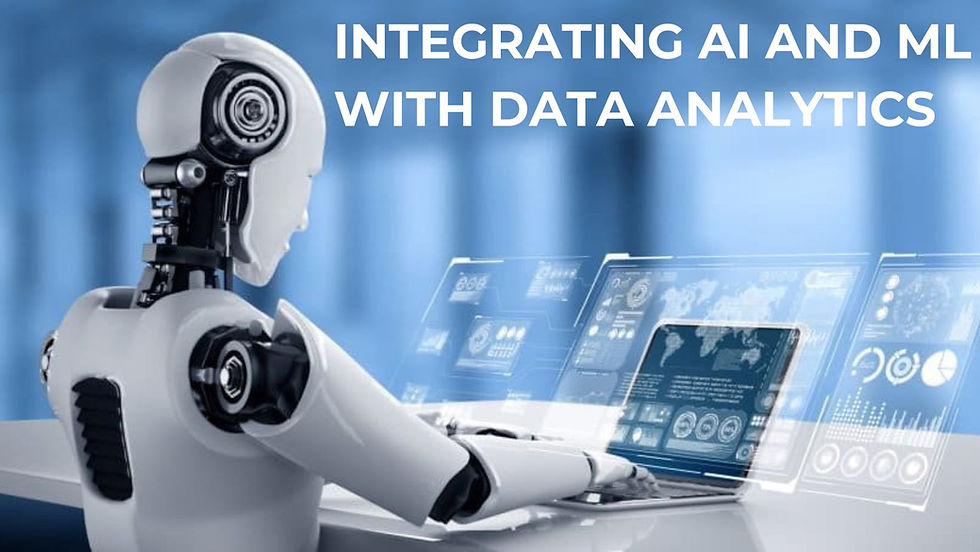
Introduction
The integration of Artificial Intelligence (AI) and Machine Learning (ML) with data analytics is revolutionizing how organizations extract insights from their data. This synergy enhances the capabilities of traditional data analytics, enabling more accurate predictions, deeper insights, and more effective decision-making. This article explores how AI and ML are transforming data analytics and the benefits they bring to various industries.
Understanding AI, ML, and Data Analytics
Artificial Intelligence (AI): AI refers to the simulation of human intelligence in machines that are programmed to think and learn. It encompasses a wide range of technologies, including natural language processing (NLP), computer vision, and robotics.
Machine Learning (ML): ML is a subset of AI that involves training algorithms on data to allow them to learn and make predictions or decisions without being explicitly programmed. It includes techniques like supervised learning, unsupervised learning, and reinforcement learning.
Data Analytics: Data analytics involves the process of examining data sets to draw conclusions about the information they contain. It includes various techniques such as statistical analysis, data mining, and predictive modeling.
Enhancing Data Analytics with AI and ML
Improved Data Processing
AI and ML algorithms can handle vast amounts of data more efficiently than traditional methods. They can quickly process and analyze large datasets, identifying patterns and trends that would be impossible for humans to detect manually. This capability is particularly valuable in industries with big data, such as finance, healthcare, and retail.
Predictive Analytics
Machine learning models excel at predictive analytics, which involves using historical data to predict future outcomes. By training models on past data, businesses can forecast trends, customer behavior, and market developments with high accuracy. This predictive capability helps organizations make proactive decisions, optimize operations, and mitigate risks.
Real-Time Analytics
AI and ML enable real-time data processing and analysis. Traditional data analytics methods often involve batch processing, where data is collected and analyzed at intervals. In contrast, AI-powered systems can analyze data as it is generated, providing immediate insights. This real-time capability is crucial for applications such as fraud detection, stock market trading, and customer service.
Automated Data Cleaning and Preparation
Data preparation is a time-consuming but essential part of the analytics process. AI and ML can automate many aspects of data cleaning, such as identifying and correcting errors, filling in missing values, and transforming data into a usable format. This automation accelerates the analytics workflow and ensures higher data quality.
Industry Applications
Healthcare
In healthcare, AI and ML enhance diagnostic accuracy by analyzing medical images, patient records, and genetic data. Predictive models help in early disease detection and personalized treatment plans, improving patient outcomes and reducing healthcare costs.
Finance
The finance industry uses AI and ML for fraud detection, risk management, and algorithmic trading. Machine learning models analyze transaction data to identify suspicious activities, while predictive analytics assist in credit scoring and investment strategies.
Retail
Retailers leverage AI and ML to optimize inventory management, personalize marketing campaigns, and enhance customer experiences. Predictive analytics models forecast demand, reducing overstock and stockouts, while recommendation systems suggest products to customers based on their preferences.
Manufacturing
In manufacturing, AI and ML improve production efficiency and quality control. Predictive maintenance models analyze sensor data from machinery to predict failures before they occur, minimizing downtime and maintenance costs.
Challenges and Considerations
Data Privacy and Security
The integration of AI and ML with data analytics raises concerns about data privacy and security. Organizations must ensure that data is handled responsibly and comply with regulations such as GDPR and CCPA to protect sensitive information.
Model Interpretability
AI and ML models, particularly deep learning models, can be complex and difficult to interpret. Ensuring that these models are transparent and explainable is critical for gaining trust from stakeholders and for regulatory compliance.
Skill Requirements
Implementing AI and ML in data analytics requires specialized skills in data science, machine learning, and software engineering. Organizations need to invest in talent development or collaborate with experts to successfully leverage these technologies.
Conclusion
The integration of AI and ML with data analytics is transforming how organizations process, analyze, and leverage data. By enhancing predictive capabilities, enabling real-time analysis, and automating data preparation, AI and ML are unlocking new insights and driving more informed decision-making across various industries. As these technologies continue to evolve, their impact on data analytics will only grow, offering even greater opportunities for innovation and efficiency. Exploring a data analytics course in Delhi, Noida or other cities across India can further empower professionals to harness these advancements and lead in the age of data-driven strategies.
API Connects is a technology services brand that supports enterprises with data, cloud, API, and AI-driven solutions, including IoT solutions and AI and ML services. Consult our engineers for digital transformation. Visit: https://apiconnects.co.nz/ai-automation-machine-learning/