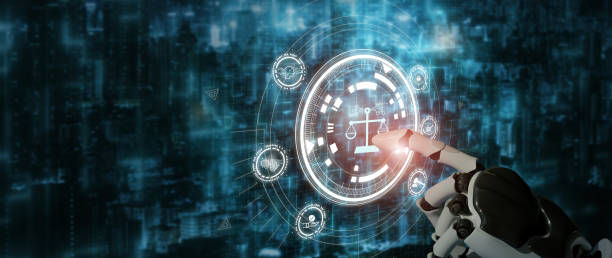
Introduction
The rapid advancement of Artificial Intelligence (AI) and data analytics is transforming industries, economies, and societies. From healthcare to finance, AI-driven data analytics offers unprecedented opportunities for innovation, efficiency, and decision-making. However, alongside these benefits come significant ethical challenges that need to be addressed to ensure the responsible use of AI technologies. This article explores the ethical implications of AI in data analytics and provides insights into how these challenges can be managed.
The Ethical Implications of AI in Data Analytics
Bias and Fairness
AI systems are only as good as the data they are trained on. If the training data contains biases, the AI will likely perpetuate and even amplify these biases. This can lead to unfair treatment of certain groups of people, particularly minorities and underrepresented communities. For instance, biased algorithms in hiring processes can lead to discriminatory practices, and biased medical data can result in suboptimal healthcare recommendations for certain populations.
Privacy and Data Security
The collection and analysis of vast amounts of data raise serious concerns about privacy and data security. Individuals' personal information can be misused or inadequately protected, leading to privacy breaches and identity theft. Additionally, the potential for AI to infer sensitive information from seemingly innocuous data poses further risks.
Transparency and Accountability
AI systems often operate as "black boxes," making it difficult to understand how decisions are made. This lack of transparency can lead to mistrust and accountability issues, particularly in critical areas such as criminal justice, finance, and healthcare. When AI-driven decisions significantly impact individuals' lives, it is crucial to ensure that these systems are transparent and that there is a clear accountability mechanism in place.
Autonomy and Human Control
AI systems have the potential to make decisions autonomously, which can undermine human autonomy and control. In scenarios where AI is used for decision-making, it is essential to strike a balance between leveraging AI's capabilities and maintaining human oversight. Over-reliance on AI can lead to complacency and reduce human agency.
Addressing Ethical Challenges in AI and Data Analytics
Implementing Fairness and Bias Mitigation Strategies
To address bias and fairness, it is crucial to implement strategies for identifying and mitigating biases in AI systems. This includes using diverse and representative datasets, employing fairness-aware machine learning techniques, and regularly auditing AI systems for bias. Additionally, involving ethicists and domain experts in the development and deployment of AI systems can help ensure that ethical considerations are integrated into the process.
Enhancing Privacy and Data Security Measures
Organizations must adopt robust privacy and data security measures to protect individuals' personal information. This involves implementing strong encryption practices, ensuring data anonymization, and adhering to data protection regulations such as the General Data Protection Regulation (GDPR). Moreover, fostering a culture of privacy and data security within organizations can help prioritize these issues at all levels.
Promoting Transparency and Explainability
Enhancing transparency and explainability in AI systems is vital for building trust and accountability. This can be achieved by developing interpretable models, providing clear documentation of AI decision-making processes, and offering explanations for AI-driven decisions. Tools and frameworks that facilitate explainability, such as LIME (Local Interpretable Model-agnostic Explanations) and SHAP (SHapley Additive exPlanations), can be instrumental in this regard.
Ensuring Human Oversight and Control
Maintaining human oversight and control over AI systems is essential to prevent over-reliance on technology. This can be achieved by establishing clear guidelines for human-in-the-loop (HITL) systems, where humans can intervene and override AI decisions when necessary. Additionally, training and educating individuals on the limitations and ethical considerations of AI can help ensure responsible use of these technologies.
Conclusion
Balancing innovation and responsibility in AI and data analytics is a complex but essential task. By addressing ethical implications such as bias, privacy, transparency, and autonomy, we can harness the transformative potential of AI while ensuring that it serves the best interests of society. It is imperative for stakeholders, including policymakers, industry leaders, researchers, and ethicists, to collaborate and create a framework that promotes ethical AI development and deployment. One way to foster this collaborative effort is through education and training.
For instance, data analytics training course in Delhi, Noida, and other locations across India can equip professionals with the necessary skills and ethical understanding to navigate the complexities of AI and data analytics. Through collective effort and continuous vigilance, we can pave the way for a future where AI-driven data analytics is both innovative and responsible.
Comentários