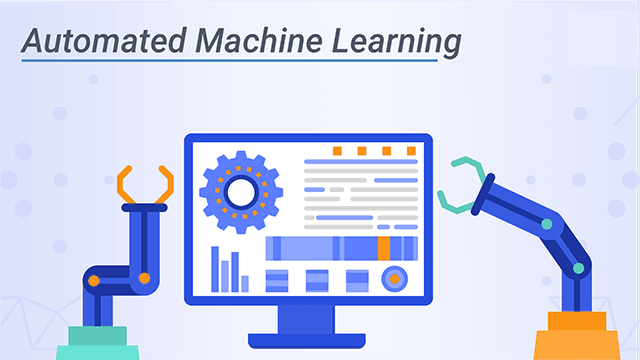
Introduction
The landscape of machine learning (ML) is evolving rapidly, driven by the increasing complexity of data and the demand for more sophisticated models. Automated Machine Learning (AutoML) is at the forefront of this evolution, promising to democratize ML by automating the end-to-end process of applying machine learning to real-world problems. This article delves into the fundamentals of AutoML, its significance, key components, benefits, challenges, and future prospects.
What is Automated Machine Learning?
Automated Machine Learning (AutoML) refers to the process of automating the tasks of applying machine learning to real-world problems. AutoML covers the complete pipeline from the raw dataset to the deployable machine learning model. This includes data preprocessing, feature engineering, model selection, hyperparameter tuning, and model evaluation.
The Significance of AutoML
AutoML aims to make machine learning accessible to non-experts and improve efficiency for experts. It reduces the time and effort required to build ML models, allowing domain experts to focus on high-level problem-solving and decision-making. Furthermore, it enables organizations to leverage machine learning without needing extensive in-house ML expertise.
Key Components of AutoML
1)Data Preprocessing: This involves cleaning, normalizing, and transforming raw data into a suitable format for ML algorithms. AutoML tools automate these steps to handle missing values, categorical variables, and data scaling.
2) Feature Engineering: AutoML systems can automatically create and select the most relevant features from the dataset, enhancing the predictive power of models.
3) Model Selection: AutoML frameworks evaluate a variety of ML algorithms to identify the best-performing model for a given task.
4) Hyperparameter Tuning: Hyperparameters significantly impact model performance. AutoML automates the process of tuning these parameters to optimize model accuracy.
5) Model Evaluation: AutoML includes rigorous model validation techniques to ensure the selected model generalizes well to unseen data.
Benefits of AutoML
Increased Productivity: Automating repetitive and time-consuming tasks allows data scientists to focus on more complex aspects of ML projects.
Accessibility: Non-experts can build and deploy ML models without deep technical knowledge.
Efficiency: AutoML optimizes the entire ML pipeline, leading to faster model development and deployment.
Scalability: It allows organizations to scale their ML initiatives by building numerous models efficiently.
Challenges of AutoML
Complexity of Real-World Data: Handling diverse and noisy datasets remains a challenge.
Interpretability: Automated models can be seen as "black boxes," making it difficult to understand their decision-making processes.
Computational Resources: AutoML processes can be resource-intensive, requiring significant computational power.
Customization: Balancing automation with the need for customization and domain-specific adjustments can be challenging.
Future Prospects of AutoML
The future of AutoML is promising, with advancements expected in several areas:
Enhanced Interpretability: Developing methods to make AutoML models more transparent and interpretable.
Integration with Edge Computing: Deploying AutoML on edge devices to enable real-time, on-device learning and inference.
Greater Automation: Further automating the ML lifecycle, including model maintenance and retraining.
Broadening Applications: Expanding the use of AutoML across various industries, including healthcare, finance, and manufacturing.
Conclusion
Automated Machine Learning is transforming the field of data science by automating complex and time-consuming tasks, making ML accessible to a broader audience. This transformation is evident in the increasing demand for data science training course in Noida, Delhi and cities acoss India, and other tech hubs, where professionals seek to leverage the power of AutoML.
While challenges remain, the continuous advancements in AutoML technologies promise a future where machine learning is seamlessly integrated into various aspects of our lives, driving innovation and efficiency across industries. As AutoML evolves, it holds the potential to democratize machine learning, making it an indispensable tool for solving some of the most pressing problems of our time.
コメント