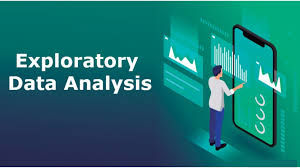
Exploratory Data Analysis (EDA) is a crucial step in the data science workflow, allowing professionals to uncover insights, identify patterns, and prepare data for advanced modeling. Whether you're a budding data scientist or a seasoned professional, EDA ensures you approach data systematically and effectively.
Here, we will delve into the eight key benefits of conducting exploratory data analysis.
What Is Exploratory Data Analysis?
EDA is a process used to summarize the main characteristics of a dataset, often with the aid of visualizations. It helps in understanding data distributions, spotting anomalies, and preparing data for further analysis. This process is an integral part of the data science journey.
Benefits of Conducting Exploratory Data Analysis
1. Identifies Missing Data
One of the primary advantages of EDA is the ability to detect missing or incomplete data. Missing values can severely impact the accuracy of predictive models, and EDA allows you to handle them proactively.
2. Uncovers Data Patterns and Trends
EDA helps analysts spot hidden patterns and trends within the dataset. For instance, it reveals seasonal trends in sales or changes in customer behavior over time, which are invaluable for decision-making.
3. Assesses Data Quality
A dataset’s quality directly impacts the outcomes of analysis. EDA helps identify data inconsistencies, duplicates, and outliers, ensuring the integrity of the data before it is used in modeling.
4. Reduces Noise in Data
By examining the dataset closely, EDA enables the removal of irrelevant variables or noisy data, streamlining the analysis process and improving the accuracy of machine learning models.
5. Enhances Decision-Making
EDA provides actionable insights that empower organizations to make informed decisions. For example, analyzing market data through EDA can help businesses identify growth opportunities.
6. Prepares Data for Advanced Analysis
A thorough EDA sets the stage for advanced techniques like predictive modeling or clustering. Clean and well-understood data ensures better results from machine learning algorithms.
7. Saves Time and Resources
By understanding data early in the analysis process, EDA minimizes errors and reduces the need for rework later. This saves valuable time and computational resources.
8. Improves Data Visualization
EDA involves visualizing data through charts, graphs, and plots, which aids in communicating findings effectively to stakeholders. Well-structured visualizations can highlight key insights clearly.
The Importance of EDA
Exploratory Data Analysis is a skill every aspiring data scientist must master. As you dive deeper into learning data science, understanding how to leverage EDA becomes essential. Institutes offering data science training course in Delhi, Noida, Faridabad, Ghaziabad, Gurgaon, Greater Noida, and other cities located in India often emphasize the significance of EDA in their curriculum. A robust foundation in EDA ensures you are well-prepared for tackling real-world data challenges.
Conclusion
Exploratory Data Analysis is a pivotal step in any data science project. By uncovering insights, ensuring data quality, and streamlining the analysis process, EDA lays the groundwork for meaningful conclusions and accurate predictions. Whether you're analyzing sales data, customer trends, or scientific research, EDA helps unlock the full potential of your dataset.
By mastering EDA, you set yourself apart as a competent data professional, ready to extract value from data in impactful ways.
Comentários